Abstract
The global energy landscape is undergoing a transformative shift toward renewable energy sources like solar and wind power. However, the intermittent and unpredictable nature of these energy sources poses significant challenges to grid stability and energy reliability. Energy storage systems (ESS) have emerged as a critical solution to address these challenges, enabling the efficient storage and dispatch of energy when needed. Yet, the economic viability of energy storage remains a pressing concern.
This is where Artificial Intelligence for Energy Storage Optimization comes into play. By leveraging advanced machine learning algorithms, predictive analytics, and intelligent control systems, Artificial Intelligence for Energy Storage Optimization can significantly enhance the efficiency, reliability, and cost-effectiveness of energy storage systems. This article explores how AI-driven solutions are revolutionizing energy storage, making it more economically viable and sustainable.
The Role of AI in Energy Storage Optimization
Enhancing Energy Management Systems
Energy Management Systems (EMS) are crucial for optimizing the operation of hybrid energy storage systems, which often combine technologies like compressed air energy storage (CAES), battery energy storage systems (BESS), and solid oxide fuel cells (SOFC). Traditional EMS rely on static models and rule-based strategies, which may not adapt well to dynamic energy markets or fluctuating renewable energy outputs. AI, particularly deep reinforcement learning (DRL), offers a more adaptive and data-driven approach.
For instance, a study by Guan et al. demonstrated how a DRL-based EMS could optimize the charging and discharging actions of hybrid energy storage systems, leading to significant economic benefits (Guan et al. 2025). By incorporating real-time data and learning from historical patterns, AI-driven EMS can make more informed decisions, reducing operational costs and improving system efficiency.
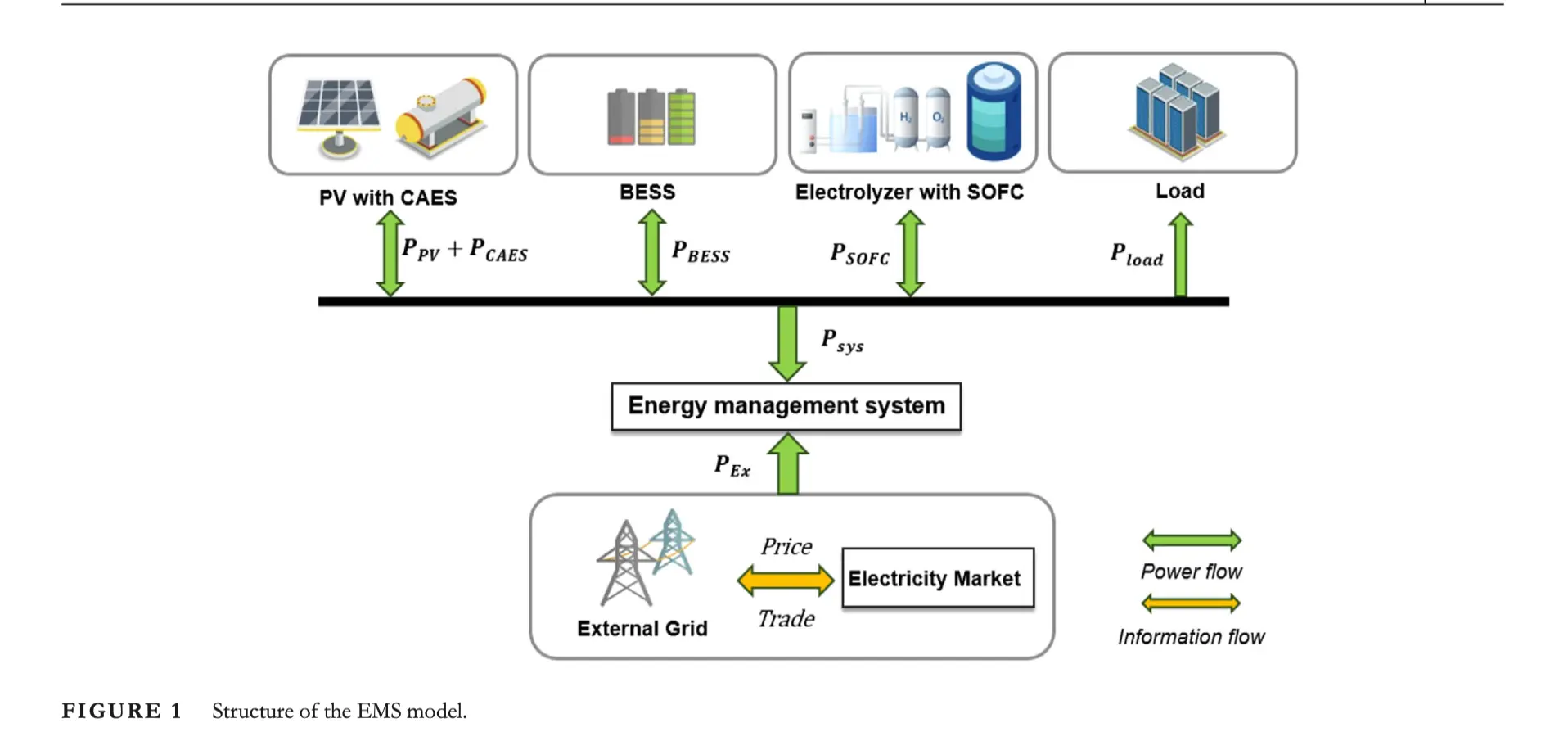
Predictive Analytics for Energy Forecasting
One of the key challenges in energy storage is accurately predicting energy demand and supply. AI-powered predictive analytics can analyze vast amounts of data, including weather patterns, historical energy consumption, and market prices, to forecast energy needs with high precision. This capability is particularly valuable for renewable energy integration, where supply can be highly variable.
For example, AI algorithms can predict periods of high solar or wind energy generation and adjust storage strategies accordingly. This not only ensures a stable energy supply but also maximizes the economic benefits of energy arbitrage—buying energy when prices are low and selling it when prices are high (Dolatabadi et al. 2023).
Intelligent Control Systems for Grid Stability
AI-driven intelligent control systems play a vital role in maintaining grid stability, especially as the share of renewable energy increases. These systems can dynamically adjust the operation of energy storage systems to balance supply and demand, prevent grid overloads, and respond to sudden changes in energy production.
A study by Huang et al. highlighted the effectiveness of AI-based control strategies in managing combined wind-solar storage systems, ensuring reliable power delivery even under fluctuating conditions (Huang et al. 2021). By continuously monitoring grid conditions and optimizing energy dispatch, AI helps maintain grid stability while reducing the need for costly infrastructure upgrades.
AI-Driven Cost Reduction in Energy Storage
Optimizing Energy Storage Capacity Planning
One of the most significant costs associated with energy storage is the initial investment in storage capacity. Overestimating or underestimating storage needs can lead to inefficiencies and increased costs. AI can optimize energy storage capacity planning by analyzing historical data, predicting future energy demands, and simulating various scenarios.
For instance, AI algorithms can determine the optimal size of a battery storage system based on factors like peak demand, renewable energy generation patterns, and market prices. This ensures that energy storage systems are neither overbuilt nor underutilized, maximizing their economic viability (Li et al. 2022).
Reducing Operational and Maintenance Costs
Artificial Intelligence for Energy Storage Optimization can also reduce the operational and maintenance costs of energy storage systems. By continuously monitoring system performance and identifying potential issues before they escalate, AI-driven predictive maintenance can prevent costly downtime and extend the lifespan of storage equipment.
Moreover, “Artificial Intelligence for Energy Storage Optimization” can optimize the scheduling of maintenance activities, ensuring they are performed during periods of low energy demand. This minimizes disruptions and further reduces costs (Sanchez Gorostiza and Gonzalez-Longatt 2020).
Enhancing Energy Arbitrage Opportunities
Energy arbitrage—buying energy when prices are low and selling it when prices are high—is a key revenue stream for energy storage systems. Artificial Intelligence for Energy Storage Optimization can enhance arbitrage opportunities by analyzing market trends, predicting price fluctuations, and optimizing the timing of energy purchases and sales.
For example, a study by Cao et al. demonstrated how Artificial Intelligence for Energy Storage Optimization algorithms could optimize the operation of lithium-ion batteries for energy arbitrage, significantly increasing profitability while accounting for battery degradation (Cao et al. 2020).
AI in Renewable Energy Integration
Facilitating the Integration of Distributed Energy Resources
The rise of distributed energy resources (DERs), such as rooftop solar panels and small-scale wind turbines, has added complexity to energy systems. Artificial Intelligence for Energy Storage Optimization can facilitate the integration of DERs by optimizing their operation and ensuring they work seamlessly with centralized energy storage systems.
For instance, Artificial Intelligence for Energy Storage Optimization can coordinate the operation of multiple DERs, balancing their output with the needs of the grid and storage systems. This not only improves grid stability but also maximizes the economic benefits of DERs (Xu et al. 2023).
Supporting Demand Response Programs
Demand response programs, which incentivize consumers to reduce energy use during peak periods, are an essential tool for managing energy demand. AI can enhance these programs by predicting peak demand periods, identifying potential participants, and optimizing the timing and magnitude of demand reductions.
By integrating AI with demand response programs, utilities can reduce peak demand more effectively, lowering the need for expensive peaking power plants and improving the overall efficiency of the energy system (Han et al. 2022).
Challenges and Future Directions
Data Quality and Availability
While AI offers immense potential for energy storage optimization, its effectiveness depends on the quality and availability of data. Inaccurate or incomplete data can lead to suboptimal decisions, undermining the economic viability of energy storage systems. Ensuring access to high-quality, real-time data is therefore critical for the success of AI-driven solutions.
Scalability and Computational Complexity
As energy systems grow in complexity, so do the computational demands of AI algorithms. Developing scalable AI solutions that can handle large-scale energy systems without compromising performance is a key challenge for researchers and engineers.
Ethical and Regulatory Considerations
The widespread adoption of Artificial Intelligence for Energy Storage Optimization also raises ethical and regulatory concerns. Ensuring transparency, fairness, and accountability in AI-driven decision-making is essential to build trust and ensure the responsible use of AI in energy storage.
Conclusion
Artificial Intelligence for Energy Storage Optimization is revolutionizing the energy storage industry, making it more economically viable and sustainable. By enhancing energy management systems, optimizing capacity planning, reducing operational costs, and facilitating renewable energy integration, AI-driven solutions are addressing some of the most pressing challenges in energy storage. As the global energy landscape continues to evolve, the role of AI in energy storage optimization will only grow, paving the way for a more efficient, reliable, and sustainable energy future.
Works Cited
Cao, J., et al. “Deep Reinforcement Learning-Based Energy Storage Arbitrage with Accurate Lithium-Ion Battery Degradation Model.” IEEE Transactions on Smart Grid, vol. 11, no. 5, 2020, pp. 4513–4521.
Dolatabadi, A., et al. “Deep Reinforcement Learning-Based Self-Scheduling Strategy for a CAES-PV System Using Accurate Sky Images-Based Forecasting.” IEEE Transactions on Power Systems, vol. 38, no. 2, 2023, pp. 1608–1618.
Guan, Y., et al. “A Learning-Based Energy Management Strategy for Hybrid Energy Storage Systems with Compressed Air and Solid Oxide Fuel Cells.” IET Renewable Power Generation, vol. 19, 2025, e13192.
Han, Y., et al. “Deep Reinforcement Learning Based Approach for Real-Time Dispatch of Integrated Energy System with Hydrogen Energy Utilization.” Proceedings of the 2022 12th International Conference on Power and Energy Systems (ICPES), 2022, pp. 972–976.
Huang, S., et al. “A Control Strategy Based on Deep Reinforcement Learning Under the Combined Wind-Solar Storage System.” IEEE Transactions on Industrial Applications, vol. 57, no. 6, 2021, pp. 6547–6558.
Li, Y., et al. “Optimal Scheduling of Isolated Microgrids Using Automated Reinforcement Learning-Based Multi-Period Forecasting.” IEEE Transactions on Sustainable Energy, vol. 13, no. 1, 2022, pp. 159–169.
Sanchez Gorostiza, F., and F. M. Gonzalez-Longatt. “Deep Reinforcement Learning-Based Controller for SOC Management of Multi-Electrical Energy Storage System.” IEEE Transactions on Smart Grid, vol. 11, no. 6, 2020, pp. 5039–5050.
Xu, T., et al. “Intelligent Home Energy Management Strategy with Internal Pricing Mechanism Based on Multiagent Artificial Intelligence-of-Things.” IEEE Systems Journal, vol. 17, no. 4, 2023, pp. 6045–6056.