Abstract
The integration of renewable energy sources, such as solar and wind, into modern power systems has fundamentally reshaped how we manage and optimize energy. However, these renewable sources are inherently intermittent and volatile, posing significant challenges to the stability and reliability of power grids. To address these challenges, Deep Reinforcement Learning in Energy Management has emerged as a groundbreaking approach, transforming traditional management systems into intelligent, adaptive, and highly efficient platforms. This innovative technology leverages machine learning algorithms to optimize energy flows, enhance grid reliability, and maximize economic returns from hybrid storage systems comprising compressed air energy storage (CAES), battery energy storage systems (BESS), and advanced technologies like solid oxide fuel cells (SOFC) (Guan et al.).
In this article, we explore how deep reinforcement learning (DRL) is revolutionizing energy management systems, highlighting recent research developments, key methodologies, and the transformative potential for sustainable energy infrastructure.
Understanding Deep Reinforcement Learning (DRL) in Energy Management
DRL (Deep Reinforcement Learning) combines artificial neural networks with reinforcement learning principles, enabling systems to learn optimal decision-making strategies through continuous interaction with the environment. Unlike traditional methods reliant on fixed rules or historical predictions, DRL adjusts dynamically, providing significant improvements in energy efficiency, cost optimization, and reliability.
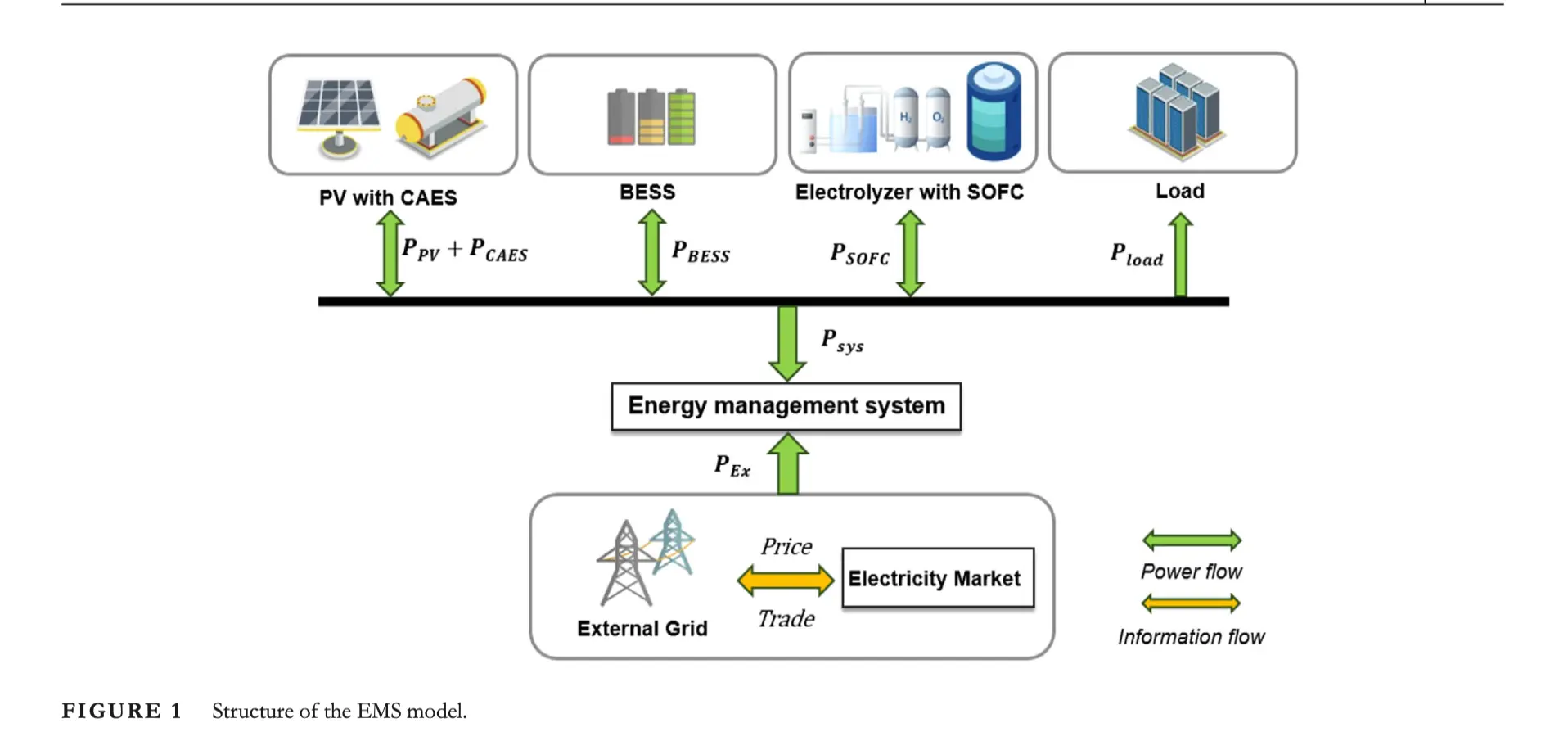
Advantages of Deep Reinforcement Learning in Energy Management Systems
According to recent studies, Deep Reinforcement Learning offers three main advantages when applied to energy management systems:
- Reduction in Modeling Complexity: Deep Reinforcement Learning reduces the need for complex physical modeling by learning directly from environmental interactions. This enables more robust energy management strategies, especially when integrating diverse resources like renewables and storage technologies (Guan et al.).
- Real-time Decision Making: Although Deep Reinforcement Learning algorithms require significant computational resources during the initial training phase, they can rapidly produce optimal scheduling decisions in real-time once training is completed, essential for managing fluctuating energy demands and renewable supply variations.
- Enhanced Adaptability: Deep Reinforcement Learning algorithms demonstrate exceptional flexibility and adaptability, capable of adjusting strategies in real-time as system conditions change, thus significantly improving the overall reliability and efficiency of energy systems (Guan et al.).
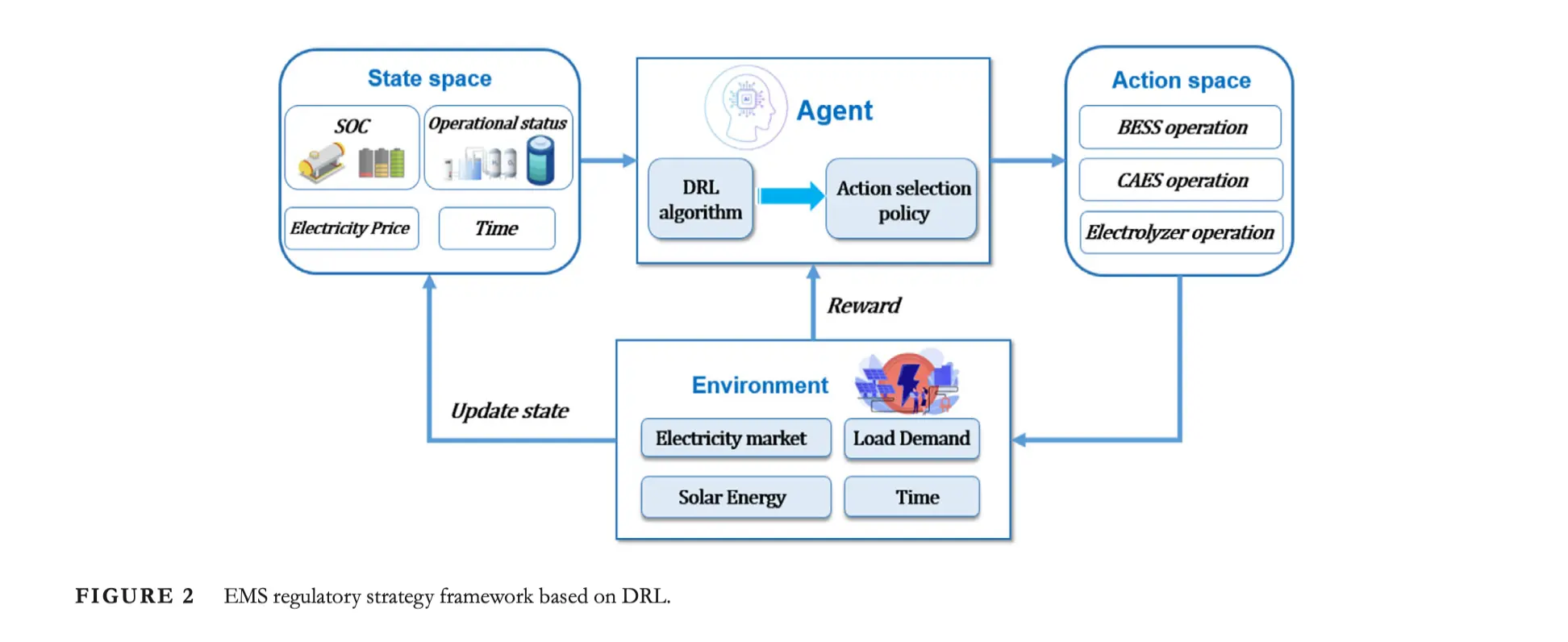
Transformative Applications of Deep Reinforcement Learning in Energy Management Systems
Hybrid Energy Storage Systems (HESS)
Hybrid energy storage systems, combining short-duration technologies such as batteries and long-duration storage methods like compressed air energy storage (CAES), are essential in maintaining grid stability. Recent research demonstrated that integrating Deep Reinforcement Learning with CAES, battery storage, and SOFCs not only enhances grid reliability but also significantly reduces operational costs and carbon emissions (Guan et al.).
The Deep Deterministic Policy Gradient (DDPG) algorithm, a popular Deep Reinforcement Learning method, has been effectively utilized for managing energy storage systems. An improved variant of this algorithm was shown to increase system profitability by accurately identifying subtle environmental changes, optimizing energy scheduling, and effectively arbitraging electricity prices (Guan et al.).
Real-Time Energy Management and Demand Response
Deep Reinforcement Learning’s ability to optimize real-time energy management makes it particularly valuable in demand-response scenarios. By accurately forecasting energy consumption and dynamically adjusting power storage and discharge schedules, DRL-driven systems can significantly enhance demand response performance and grid stability, resulting in substantial energy cost reductions and higher energy efficiency (Guan et al.).
Electric Vehicle Charging and Grid Integration
Integration of electric vehicle (EV) charging infrastructure with renewable energy sources and grid services represents another transformative application of Deep Reinforcement Learning. Smart grids leveraging DRL strategies dynamically schedule EV charging based on real-time demand forecasting, renewable energy availability, and grid constraints, significantly enhancing grid stability and reducing peak demand stresses (Guan et al.).
Real-World Case Study: DRL-Based Hybrid Storage Management with CAES and SOFC
Recent research conducted by Guan et al. (2025) explored the application of Deep Reinforcement Learning for hybrid energy storage management, integrating compressed air energy storage (CAES), battery energy storage systems (BESS), electrolyzers, and solid oxide fuel cells (SOFC). The study aimed to optimize real-time scheduling and energy dispatch while maximizing economic profitability.
The key findings include:
- Deep Reinforcement Learning algorithms effectively optimized charging and discharging schedules, considering economic parameters like electricity market prices and storage capacity constraints.
- The improved DDPG algorithm demonstrated superior sensitivity to environmental changes compared to basic Deep Reinforcement Learning approaches, leading to optimal real-time energy decisions and increased economic returns.
- Real-time management of CAES and SOFC significantly enhanced system resilience and improved profitability, highlighting the substantial potential of Deep Reinforcement Learning in real-world energy system scenarios (Guan et al.).
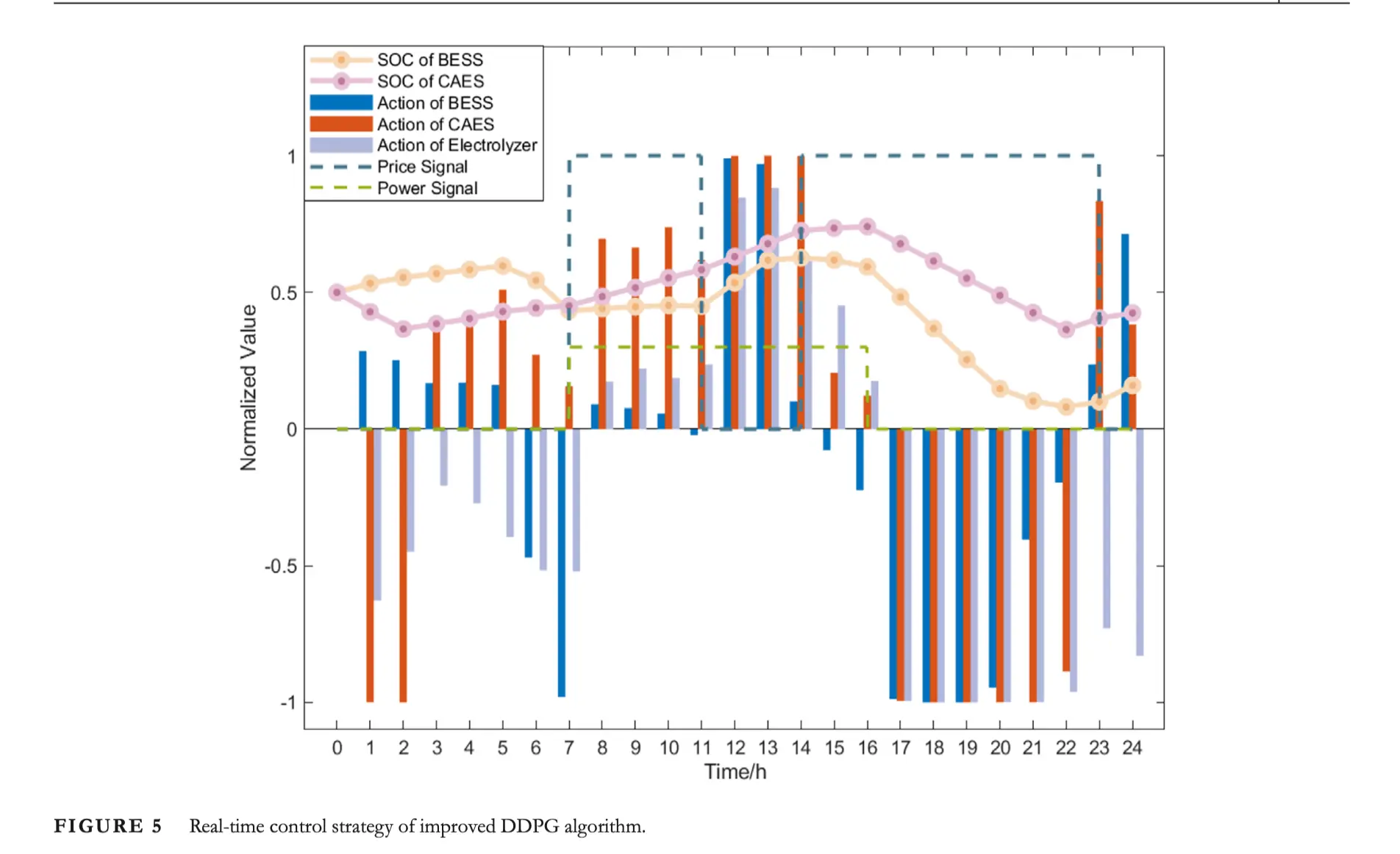
Challenges and Future Directions
Despite significant advancements, implementing Deep Reinforcement Learning in energy management systems also faces specific challenges:
- High Computational Requirements: Deep Reinforcement Learning methods require extensive computational resources for initial training, posing barriers for rapid deployment. Future developments should focus on efficient training algorithms or transfer learning techniques to reduce initial training time and computational burdens.
- Sensitivity to Parameter Tuning: DRL algorithms, including DDPG, are highly sensitive to hyperparameter adjustments. Further research is needed to develop adaptive algorithms capable of autonomous hyperparameter optimization, improving overall system robustness.
- Integration with Real-World Infrastructure: Effective integration of DRL algorithms into existing grid infrastructure, particularly legacy systems, remains challenging. Collaborative approaches between academia and industry are essential for streamlined adoption (Guan et al.).
Conclusion
Deep Reinforcement Learning in Energy Management represents a significant leap forward in optimizing energy systems and integrating renewable energy sources. By effectively managing hybrid energy storage technologies, facilitating renewable energy integration, and optimizing real-time energy dispatch, DRL methodologies have demonstrated profound potential for transforming energy infrastructures into intelligent, resilient, and economically viable systems.
Future research and development in DRL technologies will undoubtedly further drive the evolution of smarter, more sustainable, and more resilient energy systems.
References
Guan, Yundie, et al. “A Learning-Based Energy Management Strategy for Hybrid Energy Storage Systems with Compressed Air and Solid Oxide Fuel Cells.” IET Renewable Power Generation, vol. 19, no. 1, 2025, pp. 1-12, doi:10.1049/rpg2.13192.
Hemmati, M., et al. “Thermodynamic Modeling of Compressed Air Energy Storage for Energy and Reserve Markets.” Applied Thermal Engineering, vol. 193, 2021, p. 116948.
Shafiee, S., et al. “Considering Thermodynamic Characteristics of a CAES Facility in Self-scheduling in Energy and Reserve Markets.” IEEE Transactions on Smart Grid, vol. 9, no. 4, 2018, pp. 3476-3485.
Xu, T., et al. “Intelligent Home Energy Management Strategy with Internal Pricing Mechanism Based on Multiagent Artificial Intelligence-of-Things.” IEEE Systems Journal, vol. 17, no. 4, 2023, pp. 6045-6056.
Zhao, Y., et al. “Meta-learning Based Voltage Control Strategy for Emergency Faults of Active Distribution Networks.” Applied Energy, vol. 349, 2023, p. 121399.
ALSO READ: Automobile Shipping Companies: Reliable Car Transport Services for Every Need